La limpieza de datos permite a las empresas trabajar con datos reales para poder tomar decisiones importantes con respecto al crecimiento de su negocio. Automatizarlo para reducir tiempo y que los datos sean 100% fiables es el objetivo de un data cleaning efectivo.
Dicen que la figura del analista de datos es y va a seguir siendo una de las profesiones más demandadas, algo que no es de extrañar teniendo en cuenta la cantidad de datos que manejan las empresas y que vivimos en la Era del Big Data.
De los mismos dependen las decisiones que se toman a diario en una empresa y de la calidad de los mismos, que esas decisiones no les hagan perder dinero. Esta es la principal razón por la que la limpieza de datos es esencial en una empresa.
Hoy te contamos qué es y en qué consiste este proceso previo a la ETL, es decir, a la extracción, transformación y carga de datos en el sistema de gestión de los mismos de una empresa.
¿Qué es el Data Cleaning o la limpieza de datos?
La limpieza de datos o depuración de datos tiene como objetivo mejorar la calidad de los datos y que estos aporten información fiable y valiosa para la toma de decisiones de un negocio u organización.
Esa limpieza consiste en corregir datos que sean incorrectos, que estén incompletos, que puedan estar duplicados o que sean erróneos, por ejemplo, por una falta de coordinación entre datos.
El data cleaning forma parte de la gestión de datos, pero es importante hacerla antes de gestionar los mismos para la toma de decisiones. Ese manejo de datos es realizado por analistas o ingenieros de datos que no solo se encargan del estudio de los mismos, sino de su limpieza.
¿Para qué sirve la limpieza de datos?
Sin unos datos de calidad, los informes que se elaboren con ellos no serán del todo fiables y mucho menos las decisiones que se tomen al respecto. Por tanto, la limpieza de datos sirve para tener una base sólida de datos sobre la que empezar a tomar esas decisiones.
Dicho esto, una correcta depuración de datos o data cleaning ayuda al negocio a:
- Tener los datos del negocio más ordenados: ya que, a diario, las empresas recogen una cantidad ingente de datos y no siempre se recopilan de manera correcta, ni se utiliza todo el potencial de los mismos.
- Evitar errores con respecto a esos datos: a veces son pequeñas decisiones del día a día, pero que nos pueden estar haciendo perder mucho dinero.
- Mejorar la productividad: ya que al hacerse de manera regular y tener los datos ordenados, no se pierde tiempo buscando datos antiguos.
- Reducir costes: tanto en lo que respecta al punto anterior, como al hecho de que por estar revisando datos de manera constante, se detectan antes errores que puedan estar causando pérdidas a la empresa.
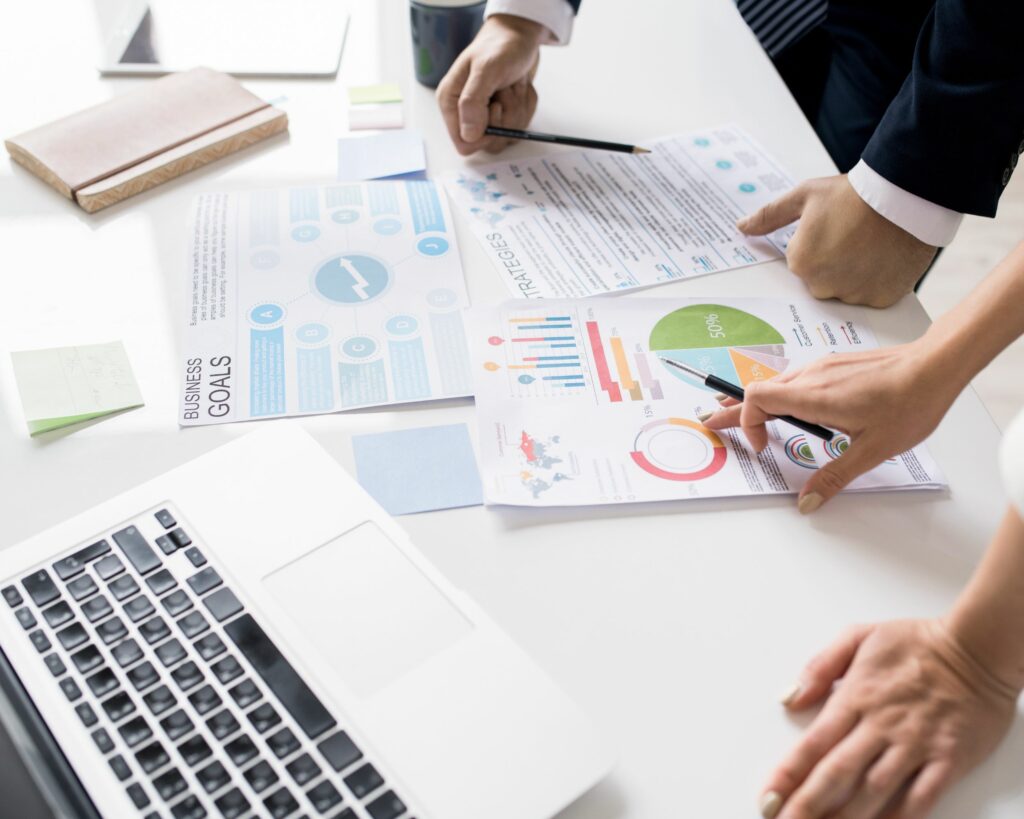
- Aumentan las ventas: gracias a la obtención de datos más fiables. Esto es algo que aprovechan especialmente los departamentos de marketing y ventas.
¿Pasos y técnicas de la limpieza de datos?
Para que la limpieza de datos sea efectiva, es importante llevar a cabo una serie de pasos previamente definidos, que serán los que nos lleven a obtener unos datos de calidad.
Esos pasos son los siguientes:
- Paso 1: Eliminar los datos irrelevantes. Tenemos mucha tendencia a querer recopilar datos en exceso y ni todos los datos que recogemos nos sirven, ni siempre tenemos los datos que necesitamos. Por esta razón, saber cuáles son los Kpi´s que nos van a dar la respuesta a las dudas que tiene nuestro negocio es el primer paso que tenemos que dar y eliminar aquellos que no nos aportan nada.
- Paso 2: Eliminar la duplicidad de datos. En ocasiones, los departamentos de análisis de datos los reciben de diferentes departamentos y llegan datos duplicados. Por eso, el segundo paso es comprobar que no haya datos duplicados. De esta forma también conseguimos que el sistema funcione mejor, al tener menos datos que analizar.
- Paso 3: Corregir los errores estructurales. Aunque la tecnología ha avanzado mucho, todavía no tienen la inteligencia de los humanos. Esta es la razón por la que es importante depurar errores como los ortográficos, pero también corregir por nuestra parte aquellos símbolos que las máquinas no siempre interpretan y que pueden dar lugar a error.
- Paso 4: Asegurarse que no faltan datos. Porque las personas que completan los datos no siempre los rellenan todos, es importante ver si faltan casillas por completar, si hay dobles espacios que estén dando lugar a datos erróneos, etc. Si vemos que hay un error que se repite siempre, deberemos retomar la estructura del formulario que se envía, de manera que podamos evitar esos errores.
- Paso 5: Filtrar datos. Con el objetivo de comprobar si hay campos de datos que podrían estar dando lugar a datos erróneos, como por ejemplo cuando se trata de hacer medias de notas, pero un solo dato puede lanzar un falso resultado o un resultado que no se corresponde con la realidad al 100%.
- Paso 6: Validar los datos. Si al principio hablábamos de eliminar datos que no aportaban nada a la empresa, en este caso hablamos de revisar si falta alguno para que nuestro negocio pueda tomar decisiones correctamente o si es necesario ajustar parámetros y añadir nuevos. Este tipo de validaciones se pueden realizar con herramientas de gestión de datos que ayuden a automatizar estos procesos, como es el caso de nuestra herramienta.
Conclusión
Toda empresa de logística y transporte que desee mejorar sus datos de negocio, mediante una toma adecuada de decisiones, basadas en datos reales y actualizados, debe pasar por un proceso de limpieza de datos de manera regular.
El mismo les va a ayudar, no solo a disponer de datos más organizados, sino a reducir costes, mejorar el rendimiento de la empresa y, por tanto, mejorar las cifras económicas.
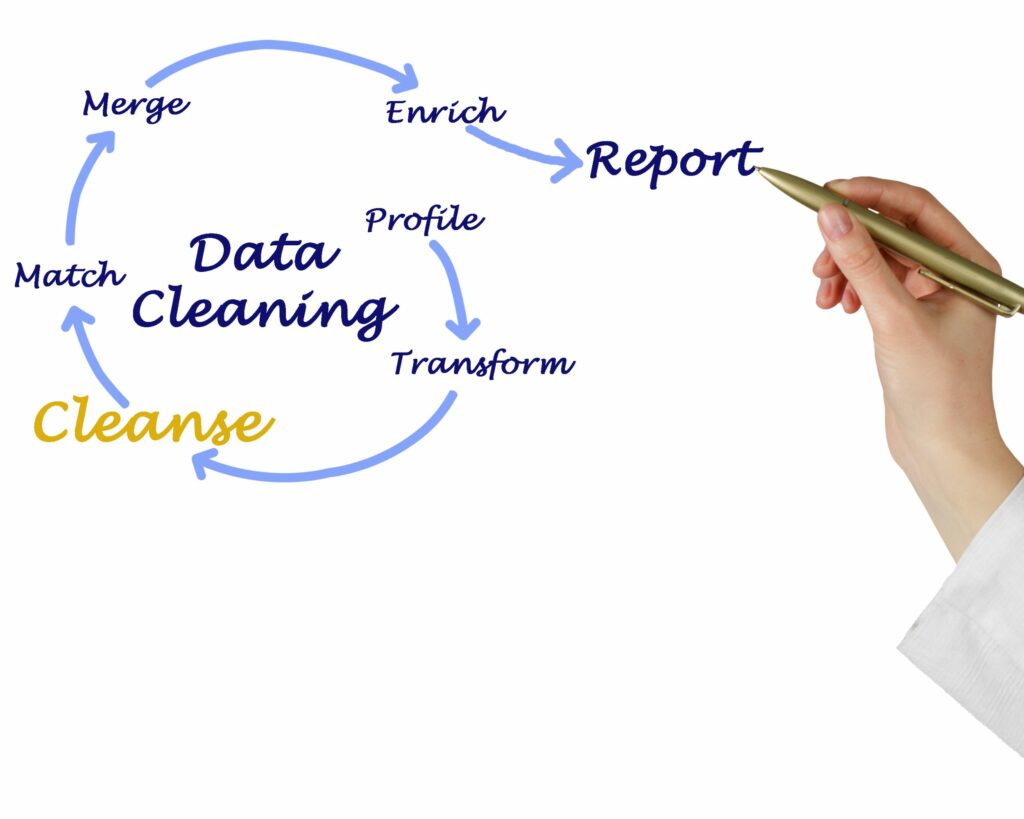